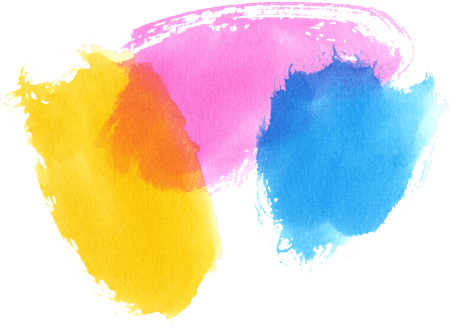
There have been many claims about technologies making healthcare better or more affordable. Artificial intelligence (AI) is one of the few innovations that can deliver on both these promises.
In this series of articles, I look at how AI can improve healthcare systems, without compromising on quality and costs. First, I reviewed the current impact of AI applications. Then, I outlined the most promising use cases for the near future.
The series ends with this article on weighing the AI benefits against financial incentives.
The biggest challenge to AI adoption
The main barrier to AI adoption in clinical practice is the limited examples of return on investment. Healthcare providers must justify using their budget to buy an AI solution. It is also an essential step to getting any innovative tool to help patients.
The medical AI industry needs evidence of a positive business case, showing cost-effective improvements. Health economics and further clinical validation studies would further support this case and increase confidence in the real-world value of AI solutions. It’s no easy task. In the UK as an example, the NHS buyer’s guide to AI in health and care provides little guidance on what makes a credible business case. The suggestion is to test hypotheses on historical data and do a pilot.
For medical imaging AI, it gets even more challenging. If you just looked at the radiology department, AI helps radiologists read and report faster on their scans. The business case is thus one of efficiency only. Yet the quality improvements that AI provides may have a major impact down the care pathway.
Hugh Harvey, Managing Director at Hardian Health, made a good point at the British Institute of Radiology (BIR) 2020 Congress:
”Radiology is not standalone. Almost all departments use radiology, so the return on investment may not be only in radiology. Health economics studies must be across departments and stakeholders.”
How to define and prove the return on investment for medical imaging AI? That is the biggest challenge for our field today. Without claiming to have figured it all out, we have a three-step approach to the question.
1. Understand the incentives of the healthcare system
The relevance of any business case depends on the healthcare system to which it applies. Healthcare systems in different countries have different payment actors, structures, and buying incentives. I will discuss three examples, simplified to keep this article concise.
The Netherlands
In the Netherlands, we identify three main possible buyers for medical imaging AI.
Dutch hospitals get a government-fixed yearly budget to cover a specific number of diagnoses and treatments. Good reasons for them to buy an AI solution are to save on costs (do more with their budget) and improve the quality of their care (which contributes to the hospital’s reputation). The solution should allow the current radiology workforce to report on more scans, with fewer errors.
Radiologists are a second buying actor. In many hospitals, they act as an independent association and may decide to buy new software to improve their budget by assessing scans faster. An AI system that automates the more tedious tasks and boosts confidence in their follow-up decisions offers a good incentive.
Thirdly, health insurers play a part, with similar goals as hospitals. Improvements in the quality of care are arguments for them to invest in AI. For example, an AI tool that supports early cancer detection would lower treatment costs, from which the insurer benefits.
The United Kingdom
Here we find a very different healthcare business model. The National Health Service (NHS) consists of separate systems for each country, and each must justify its budgets. But overall, efforts to improve healthcare are centralised. The NHS drives the adoption of and pays for new technologies.
The NHS is an evidence-based system and will look at cost-effectiveness downstream. For example, by considering the value of life year (VOLY): the monetary value for the society of a person living a year in perfect health. Or the cost of disease: lung cancer in the UK is the most expensive, evaluated at 2.4 billion, because of low survival rates and high care costs. Improving lung cancer prognosis is thus an incentive for the NHS to finance technologies such as ours (through the new AI award).
The adoption of new technology in the National Institute for Health and Care Excellence (NICE) guidance is also an argument for the NHS to support its implementation. NICE is sponsored by the Department of Health and Social Care to give evidence-based advice on health improvements.
On a hospital level, the return on investment in new technology is a crucial factor for procurement. One reason to buy AI would be to prevent financial penalties for not meeting NHS’ performance targets (e.g. targets for cancer waiting times). Tools that bring both efficiency and quality gains to this service are useful.
UK radiologists working for the NHS do not have a commercial incentive or budget to buy a new medical solution. Nonetheless, they may ask the hospital to consider software that reduces their workload.
The United States
The US is an interesting case. Recently, we saw the first government reimbursement for a radiology AI system. The reimbursement is approved by the Centres for Medicare & Medicaid Services (CMS). This breakthrough is well explained in an article by Dr Oakden-Rayner.
The US healthcare system is a combination of public funding (e.g. Medicare via the federal government, and Medicaid via states) and private management and delivery (through Healthcare Management Organisations or HMOs, groups composed of hospitals, doctors, and insurance companies). These programmes blend in pretty much an all-in-one system. We can argue that, if technology improves efficiency or accuracy for the care provider, the payor also benefits. It is a market we look forward to exploring soon.
HMOs can have different incentives. For profit-driven HMOs, AI solutions can drive more patients through their systems. Non-profit HMOs might benefit from AI that reduces care costs for patients within their system.
Despite the different setups, US radiologists, employed by the HMOs or independent practices, have similar incentives to European physicians. With one addition: to lower the risk of error and possible lawsuits, a more common practice in the US.
2. Define the AI’s benefits – the Veye Lung Nodules example
Before approaching any healthcare system, the main benefits of an AI clinical application should be clear. Here is how we look at the possible impact of using our pulmonary nodule management solution, Veye Lung Nodules:
The direct impact: faster reading and reporting
Radiologists reading a chest CT in routine clinical practice must manage the pulmonary nodules within the scan. This task involves detecting, classifying, and tracking how the nodules may evolve. Veye Lung Nodules can save time as a result of the following features:
Faster detection
Veye Lung Nodules automatically detects pulmonary nodules as small as 3mm on chest CTs. Reporting on routine scans may take less time when using it as an assistant.
Automated prior study analysis and comparison
A radiologist would (often manually) look up the prior, measure the pulmonary nodules, compare them, and calculate the volume doubling time (VDT). Veye Lung Nodules retrieves the most recent prior and returns the VDT per nodule, potentially speeding up the process.
We have plenty of anecdotal evidence of time savings using Veye Lung Nodules. Yet, organising studies is challenging. Performing one at scale in clinical practice would burden the day-to-day workflow. Still, we have started studies in the Netherlands and will do the same in the UK this year (as part of the AI award), so we are going to back up our anecdotal evidence.
The indirect impact: prevention of late-stage diseases
Using Veye Lung Nodules, indirect improvements would be:
Detecting more actionable nodules
Veye Lung Nodules may increase the radiologist’s accuracy in detecting potentially threatening pulmonary nodules. During prior analysis, nodule growth might be underestimated due to reliance on diameters instead of volume measurements. Within the first 75 cases at the first hospital where we installed our solution, Veye Lung Nodules detected a growing nodule which four radiologists had missed. Immense impact on the patient!
Reducing unnecessary follow-ups
If a lung nodule seems to be growing because the diameters are skewed or incorrectly measured, it might be deemed malignant while it is not. Veye Lung Nodules’ precise measurements might reduce the need for a biopsy, together with risk and anxiety for the patient.
3. Match the benefits with the incentives
To come to a business case, match the benefits of the AI application with the local incentives and stakeholders. For example, in the Netherlands, a focus on the return on efficiency makes a relevant case. In the UK and the US, both efficiency and quality gains can support a buying decision, with quality being particularly relevant for national health systems and HMOs.
Below, I share some practical insights into how we build our business case for Veye Lung Nodules. Of course, the benefits we present are weighed against the costs of the solution.
Efficiency gain
Based on user testimonials (clinical studies pending), we can estimate the time saved using Veye Lung Nodules as a second or concurrent reader. Our estimates are across:
- Chest CTs scenarios: without lung nodules, with lung nodules, and with lung nodules and prior studies. The time saving is highest with the latter.
- The four clinical features of Veye Lung Nodules: detection, classification, quantification, and growth assessment.
To build a case for efficiency gain, we begin our analysis at the study level and work our way up to the hospital level. We focus on the hospital-specific figures, with figures such as:
- The scan reading cost per minute (deduced from the average salary of a radiologist)
- The total number of chest CT scans the hospital performs per year
- The hospital’s estimated percentage of scans that contain lung nodules
- The percentage of the scans with lung nodules where a prior is also available
Our business case model presents the reading time for each scenario with and without Veye Lung Nodules. The resulting difference in time translates into cost savings. We then extend the findings to the total scans in a year, breaking them down per scenario and feature. Finally, we calculate the total potential savings per year.
Quality gain
A simplified way to look at the quality gain is by estimating how many actionable nodules were missed by radiologists. Malpractice cases have been reported, mostly due to recognition errors. Based on the hospital’s total number of chest CTs and local clinical studies, we can estimate the number of overlooked early-stage lung cancers.
If a well-performing AI algorithm spots these easy-to-miss early-stage lung cancers, later-stage treatments may no longer be necessary. The financial consequences are significant. Treatment costs differ for the different stages (here is an example from the Netherlands). A recent US-based study estimates 25% cost savings from early diagnosis.
Furthermore, AI-aided physicians may detect lung cancer at an early stage, and subsequently cure it. Each year added to the patient’s life has value (should no other health issues occur).
Bring it all together
To sum up, all stakeholders benefit from efficiency gains. Quality gains are only relevant for payors like insurers, national health systems and HMOs looking to reduce costs. In a simple table, the business case follows these lines:

The biggest impact of AI clinical applications will be downstream, on the treatment costs. Technology like Veye Lung Nodules can potentially reduce cancer treatment costs by tens of thousands of euros per year per hospital by detecting one or two nodules the radiologist alone would have missed. We, as an industry, need to develop clear evidence of this impact.
For now, hospitals and practices are purchasing our solutions using their operational budgets. They base their decision on the positive experience of reference sites, both with workflow and patient impact.
Once we have demonstrated cost-effectiveness, insurers and payors will have the incentive to reimburse AI tools. Then, every hospital will be able to adopt them.