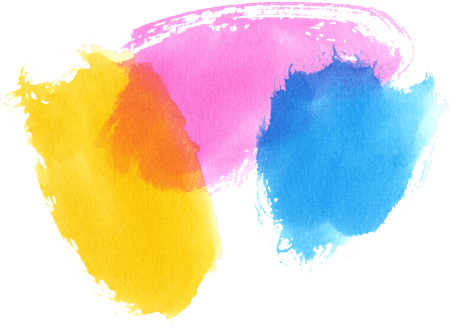
Last year, I felt like I’d let people down after deciding to leave my clinical radiology training post. Recently, however, I found myself proud and excited to have the opportunity, with my current role, to support radiologists involved in what I believe could greatly improve people’s lives: lung cancer screening programmes.
End of January, I stood in front of an audience of healthcare practitioners at a conference hosted by the British Institute of Radiology, talking about the work we are doing to support NHSEs targeted lung health checks. Beyond the proven benefits of screening initiatives, I feel we should also focus on improving the work-life of radiologists, and I highlighted ways to do that in my talk.
In this blog series, I have gathered ideas and information about the main developments in lung cancer screening (part 1) and built a case on how AI can make it successful (below). I understand that screening may continue to be delayed due to the ongoing covid-19 pandemic, but it remains important to plan these programmes for their (re-)start at the end of this year or early 2021.
Radiology as the starting point
Imaging plays a fundamental role in lung cancer screening programmes. So, when it comes to improving technology to support the programmes, the radiology department is a good place to start.
The goal of screening is to pick up early cancers which can be treated and potentially cured, therefore improving patient outcomes (as outlined in my previous blog and the NHSE long-term plan). Low-dose CT has been shown to provide sufficient image quality for the detection of early disease, whilst minimising radiation dose in asymptomatic individuals. Thoracic radiology expertise is required to determine which lung nodules may be malignant and therefore require invasive investigation, and which are likely benign and can be monitored with intermittent imaging. Appropriate follow-up recommendation helps avoid unnecessary invasive procedures, such as biopsies, and minimise patient anxiety. Both are important measures of the efficacy of lung cancer screening programmes.
End-to-end lung cancer screening involves input from many healthcare professionals. Thus, intelligent computer systems across specialities would benefit multidisciplinary teamwork. Thus, beyond image analysis, there are many opportunities for technology to add further support for effective and sustainable screening programmes. For instance, it could aid in the optimisation of image acquisition, access to imaging reports and relevant clinical details, tracking patient follow-up, or communication between patients and GPs.
Where AI-based image analysis makes a difference
Reading and reporting CT scans is time-consuming, and within a workforce already under strain, introducing a new CT-screening programme seems like a tall order. AI-driven solutions can support radiologists and contribute to successful lung cancer screening by bringing improvements in three areas:
1. Performance
Computer intelligence can increase the performance and productivity of CT reporting, freeing up time for radiologists to spend on clinical decision-making and complex cases. Specifically, AI software is well-suited for precise:
- Detection of elusive lung nodules, and differentiation of subtle changes;
- Automatic volume measurements, to help determine the appropriate frequency of monitoring (e.g. stable vs growing nodule, according to the BTS guidelines).
What further distinguishes computers from humans is the absolute consistency in their high performance, without being impacted by common external stressors to which a radiologist would be exposed (e.g. time pressure, workload, interruptions).
2. (E)quality
Having a ‘second pair of eyes’ looking at the scan can increase the confidence of the radiologist in their own assessment. Additionally, making AI-driven, accurate measurements available regardless of the level of expertise of the reporting radiologist could not only benefit quality assurance but also equality within the radiology department. The use of AI would reduce the need for all scans to be reported by the most experienced thoracic radiologists with an interest in early lung cancer detection, and instead facilitate spreading the workload across the workforce.
Another use case concerns quality assurance when outsourcing to teleradiology companies. AI-based image analysis can improve the consistency of reporting, drive the recommended terminology use, and, essential for lung cancer screening, ensure access to relevant prior imaging for comparison and change assessment over time.
3. Efficiency (via integration)
An intelligent computer system should not slow down reporting turnaround times, but improve efficiency, as well as quality, to ultimately minimize time to diagnosis (for example, the NHSE long-term plan introduces a 28-day standard from referral to diagnosis or rule out).
Older CAD technology was often described as ‘clunky’ – requiring images to be uploaded to separate systems for analysis. Additional manual steps between image acquisition and the radiology report make the process time-consuming, and often require radiology support staff to manage the workflow. It is important to consider allocative and technical efficiency, which play important roles in the evaluation of screening programmes, and their impact on healthcare systems.
An AI-driven image analysis software that is fully integrated into the radiologist’s pre-existing workflow can provide automatic results, without needing additional departmental resources. An additional benefit of fully integrated AI solutions is that their use is not restricted by time or place, therefore supporting flexible and remote working. In the context of the covid-19 pandemic, it’s been encouraging to see the increase in remote reporting, whilst maintaining a functioning department, in many hospital trusts. Going forward, it will be interesting to see whether radiologists will have the option to continue to work remotely where possible.
Valuing input from healthcare professionals
New lung cancer screening programmes will be monitored regularly to evaluate their effectiveness and determine areas for review. Commitment from all parties to work together will facilitate the optimisation of the pathway to achieve better patient outcomes and positive impacts on healthcare systems.
In our experience, close collaboration between MedTech and healthcare professionals is important for learning lessons along the way. Understanding radiologists’ needs helps tech teams develop a clinically valuable tool.
For example, our interactive lung nodule reporting tool, Veye Reporting, was designed based on the needs of radiologists involved in reporting lung screening scans. From our conversations with them, we understood that following the detailed and complex reporting protocols in lung cancer screening programmes make for labour-intensive, repetitive tasks.
To help them produce reports that follow the standardised NHSE proforma and facilitate audit for quality assurance, we added Veye Reporting as a feature to Veye Lung Nodules, focusing on making it easy to use and efficient. With this tool, the radiologists further have control over which nodules to include in the report, different sharing options, and the choice to add incidental findings.

What’s next?
Cancer services have been impacted by the covid-19 health emergency. In the UK, screening has been paused and planning to (re-) start at the end of 2020 or the beginning of 2021. Talks of introducing screening are ongoing in various European countries. However, so are concerns about catching up with the backlog of screening scans.
The British Society of Thoracic Imaging and the Royal College of Radiologists released these considerations for optimum lung cancer screening roll-out over the next five years. Their statement below is a reminder of why it is worth overcoming challenges and leveraging technology to make screening programmes a success:
